Data Science Decoded
We discuss seminal mathematical papers (part of them are really old 😎 ) that have shaped and fundamentally established the fields of machine learning and data science as we know them today. The goal of the podcast is to introduce you (and us) to the evolution of these fields from a mathematical and sometimes slightly philosophical perspective. We will discuss the contribution of these papers, not just from a pure mathematical aspect but also how they influenced the discourse in the field, which areas were opened up as a result, and so on. Our podcast episodes are also available on our youtube channel: you are invited to listen, subscribe, follow, and most importantly, correct the mistakes we have probably already made and will make in the future: https://youtu.be/wThcXx_vXjQ?si=vnMfsddZGq3MsaJ2 Our podcast website: https://datasciencedecodedpodcast.com/
Episodes
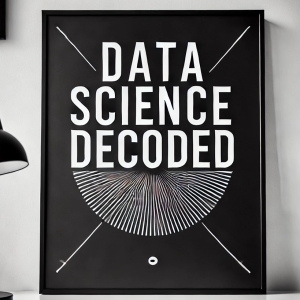
Wednesday Jun 26, 2024
Wednesday Jun 26, 2024
We discuss Ronald A. Fisher's paper "On the Mathematical Foundations of Theoretical Statistics"(1922), which profoundly shaped the field of statistics by establishing key concepts such as maximum likelihood estimation, which is crucial for parameter estimation in statistical models.
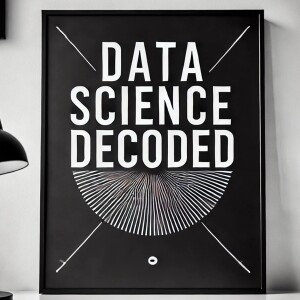
Monday Jul 01, 2024
Monday Jul 01, 2024
 "Application of the Logistic Function to Bio-Assays" (1944), Berkson Joseph
Â
The logistic function has its roots in the early 19th century, introduced by Belgian mathematician Pierre François Verhulst in 1838.
Â
It gained further prominence in the 20th century through applications in various fields, including biology and bio-assay. Joseph Berkson's 1944 paper, 'Application of the Logistic Function to Bio-Assay,' was pivotal in popularizing its use for estimating drug potency. Berkson argued that the logistic function was a more statistically manageable and theoretically sound alternative to the probit function, which assumed that individual susceptibilities to a drug follow a normal distribution.
Â
The logistic function's ability to be easily linearized via the logit transformation simplifies parameter estimation, making it an attractive choice for analyzing dose-response data.

Data Science Decoded(Fully)
The Data Science Decoded Podcast explores seminal mathematical papers that have significantly influenced the fields of machine learning and data science.
The podcast aims to bridge historical mathematical methods with modern practices, emphasizing the evolution of statistical concepts and their impact on contemporary data science.
Hosted by experienced professionals, Daniel Aronovich, Dr. Mike Erlihson and Dr. Nir Regev, the episodes provide insights into the foundational works and their relevance to current methodologies. The content is available on various platforms, including Spotify, Apple Podcasts, and YouTube.
For more information, visit [Data Science Decoded Podcast](https://datasciencedecodedpodcast.com/).